Measuring success
Designers, it's just as important as knowing Figma
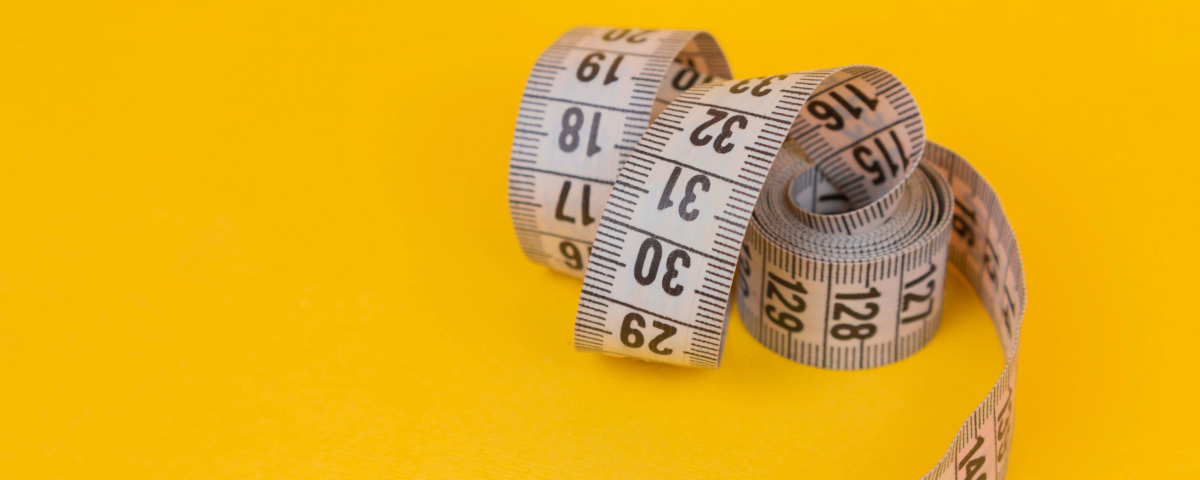
Image credit: Unsplash
Most portfolios I see check all the boxes: research, persona, user journeys, diagrams, wireframes, prototypes… But way too many of them are missing the most important piece: measuring success.
That’s a red flag, and ironically, not because the actual numbers matter, but rather because it gives the impression that the designer doesn’t understand the business they’re designing for.
Measuring success is one of the key areas of what is now becoming known as “growth design”. In short, a growth designer seeks to help a company achieve long-term growth by studying and experimenting with user behaviour across a product. It’s a data-driven, iterative approach to optimizing the user experience.
But wait, I’m not a growth designer! Why should I care?
Well I’m not a visual designer, but I still know how to make decent looking screens in Figma. And while I understand there are many other fascinating areas of design you can specialize in, not having any growth design skills is like me saying I only know how to make wireframes. This, like I’ve mentioned before, is because our role as designers is to drive a business forward, and we need to be able to understand if our work is accomplishing that.
And by the way, visual design also has an impact on user behaviour, since the Aesthetic-Usability Effect tells us that people perceive visually pleasing products as more usable. So why not use data to hone your visuals and grow your user base?
Anyways, let’s dive in.
How to optimize an existing product with experiments
Experiments enable us to test small design changes in order to optimize an existing product or feature. Those changes are rooted in research by continuously studying user behaviours.
I highly recommend reading Richard Yang’s article to dive deeper into the process of running an experiment. But in short, an experiment is comprised of the following:
- Business goal: your “why”. Do you want to generate more checkouts? Acquire more users? Retain more users? Grow your profit margin? The business goal is what drives you to design in the first place, and you should never take your eyes off it.
- Hypothesis: your “what”. A hypothesis has a clear structure: If [independent variable, i.e. the design change], then we will [dependent variable, i.e. business goal], because [assumption]. As an FYI, Brian Chesky recently spoke about how every design project at Airbnb starts with a hypothesis.
- KPIs: your “how”. KPI stands for Key Performance Indicator. These are the metrics you’re going to be tracking that will indicate whether your hypothesis is true or false, and whether your design is helping you achieve your business goal. Examples of KPIs are conversion rates, click-through rates, retention rates, average order value… You get the picture.
When working on an experiment, I always write the above three points in my Figma file in a LARGE font, and keep coming back to it throughout my design process. It helps prevent me from going astray, which let’s be honest is ridiculously easy when you’re deep into designing.
Anyways, here’s an example of an experiment on a e-commerce website:
- Business goal: increase average order value (AOV)
- Hypothesis: if we recommend complementary products during checkout, then we will increase average order value, because customers don’t always think of complementary products when shopping
- KPIs: AOV; Conversion from Checkout Launched to Checkout Completed; Average number of items in basket
So how do you measure success?
Technically, not every experiment requires an analytics tool to measure success, but unless you’re a brick and mortar business, you’ll probably need one. The easiest (and freest) tool out there is Google Analytics, which is fairly simple to set up. You’ll want to be mindful in creating “events” (ex: Viewed Product) and setting up a “funnel”, which is a sequence of events (ex: Viewed Product -> Started Checkout -> Completed Order).
More advanced tools obviously offer a greater set of features than Google Analytics, such as specific user tracking, real-time data and more. But you don’t need to worry about that if you’re just starting out.
Picking the right type of experiment
Data never lies, but it can be deceiving if you take it at face value. You have to have enough data to reach Statistical Significance, which basically tells you whether the results of test are reliable or just a stroke of luck. In order to reach statistical significance, you need to have enough traffic to your product.
If you have a decent amount of traffic, the clearest way to test a design change is by launching an A/B test. This is when you split your user base into 2 groups: a control group, where users will see version A (the current design), and the treatment group, where users will see version B (the design your testing). You measure KPIs on each version, compare which one performed better, and deploy that design to all users moving forward.
Rather than showing you examples of A/B tests here, I encourage you to check out abtest.design by Mobbin, which has a wide variety of examples of A/B tests by the likes of Headspace, TikTok, Github and more.
An important note about A/B tests is that you need to limit your experiment to a single, precise change. Sure, you can combine multiple changes in version B (ex: changing the button’s call-to-action AND its position) and see if it performs better than version A, but how will you know which of these changes actually had an impact on the results? What if one of your changes had a positive impact while the other didn’t? If you want to test multiple changes at once, you can create different combinations of changes and use multivariate testing, which will determine which combination yields the best results.
With that said, conducting A/B or multivariate tests is costly, and it can take several weeks to implement and obtain results. Not only that, if you don’t have high traffic on your product yet, it will take you an exorbitant amount of time to reach statistical significance. Most companies can’t afford to sit around and wait that long.
If this is your case, try shipping your new designs and compare results with prior metrics. This helps you gather data faster since you’re not splitting your traffic, and requires significantly less resources to implement. With that said, you have to be hyper-aware of external factors, also known as confounders, that could influence the outcome of your change. For example, running a test like that at Clutch is tricky since data naturally fluctuates with the conditions of the Canadian used car market. Similarly, if any commerce business deploys a new design on Black Friday, their data will be skewed.
Lastly, if you don’t have the resources to perform an A/B test, AND encounter too many confounding factors to get unbiased results from an experiment, you can always fall back on a good ole fashioned usability test. Qualitative data is still very telling, and while you won’t be able to get a fully accurate read on the impact of your design, you will most likely tell whether the impact is positive or negative.
But I’m designing a completely new product, what is there to measure?
The answer is simple: everything. You’re looking at a blank canvas, and you can’t imagine all the marvels that lay ahead. No but seriously, you really can’t. Like, you need data.
Jokes aside, you need to start measuring user actions early on for a few reasons:
- First, this is how you’ll identify growth opportunities in your product as your traffic increases. When you look at a funnel, where do most users drop-off? Has there been a spike in conversions? Does a specific entry point yield better results than another (i.e. one landing page vs another)? Not only do you need to keep a holistic view of your users on a continuous basis, you also need to interpret results and iterate on them.
- Second, this will give you a benchmark to measure against when you iterate on your designs. If you don’t have the budget to run an A/B test as mentioned above, you still need to analyze the impact of changes you make. For this, you need to know how your designs performed before you pushed any changes.
Some common pitfalls to avoid
- Confirmation bias: in the context of measuring success, “confirmation bias” is the tendency to emphasize metrics that suggest your design works well while ignoring the data that reveals issues in your work. One way to avoid this is to set expectations with your team early on that your experiment might very well fail, but that either way you will come out with a win. Because of confirmation bias, we tend to either claim victory too soon and stop a test when the results are what we’d like them to be, or look for explanations when the data isn’t favourable. It’s important to remember that our experiments are rooted in assumptions, and that it’s better for our test to fail than to keep a design that hurts our user’s experience.
- Looking at micro conversions only : Remember the funnel? A micro conversion is the conversion rate from one step of the funnel to the next. A macro conversion is the conversion rate of the full user journey that achieves your business goal. For example, if you are working on an e-commerce site and made a design change to the Product Page, your micro conversion would be from “Product Page Viewed” to “Checkout Launched”, while your macro conversion would be from “Product Page Viewed” to “Checkout Completed”. The reason it’s important to look at both conversions is that an increase in your micro conversion doesn’t always translate in an increase to your macro conversion. In fact, it might do the very opposite and hurt the business. Looking at both micro and macro conversions will ensure you keep a holistic view of your product and understand the true impact of the design you’re testing.
- Overlapping experiments that blur results: The same way you need to limit your test to a single change on a screen, you also need to limit the number of tests on a funnel. Your micro conversions might be independent, but your macro conversion will be conflated, and you won’t be able to tell how each test affect it.
- Remember the user: never forget that whatever your north star, it’s ultimately driven by the user. Don’t let growing metrics come at the expense of the user experience.
I don’t have any data for my portfolio. What should I do?
I’ve spoken to quite a few designers who have this issue. Some will argue I shouldn’t be telling you this, but oh well f*** it:
- It’s ok if you don’t have the exact data on hand from a case study, you should just include whatever metrics you have (or recall). Hiring managers don’t care about the exact numbers. They just want to know 1) what you measured, 2) how you measured it, and 3) what you did with the results. Even showing that you understood why an experiment failed is a plus (at least in my book)
- If you didn’t get to measure at all because you handed off designs to a client and didn’t get to continue working on the product, then include 1) what you would have measured, 2) the different possible outcomes you’d expect, and 3) how you would have used design to address each outcome
Conclusion
The beauty of product design is that unlike so many creative fields, success is not subjective. It’s tangible. Trust me, after spending 10 years in the music business where the approach is to “release and hope it works”, I’ve developed immense appreciation for the tools we have at our disposal to tell us whether a product is working as intended or not. Sure, it’s easier to pretend like everything we create is grandiose, but having actual data to back up the impact of our work on users and businesses is one of the most rewarding feelings I personally find in the field of design.